DATA QUALITY by QUANTUM
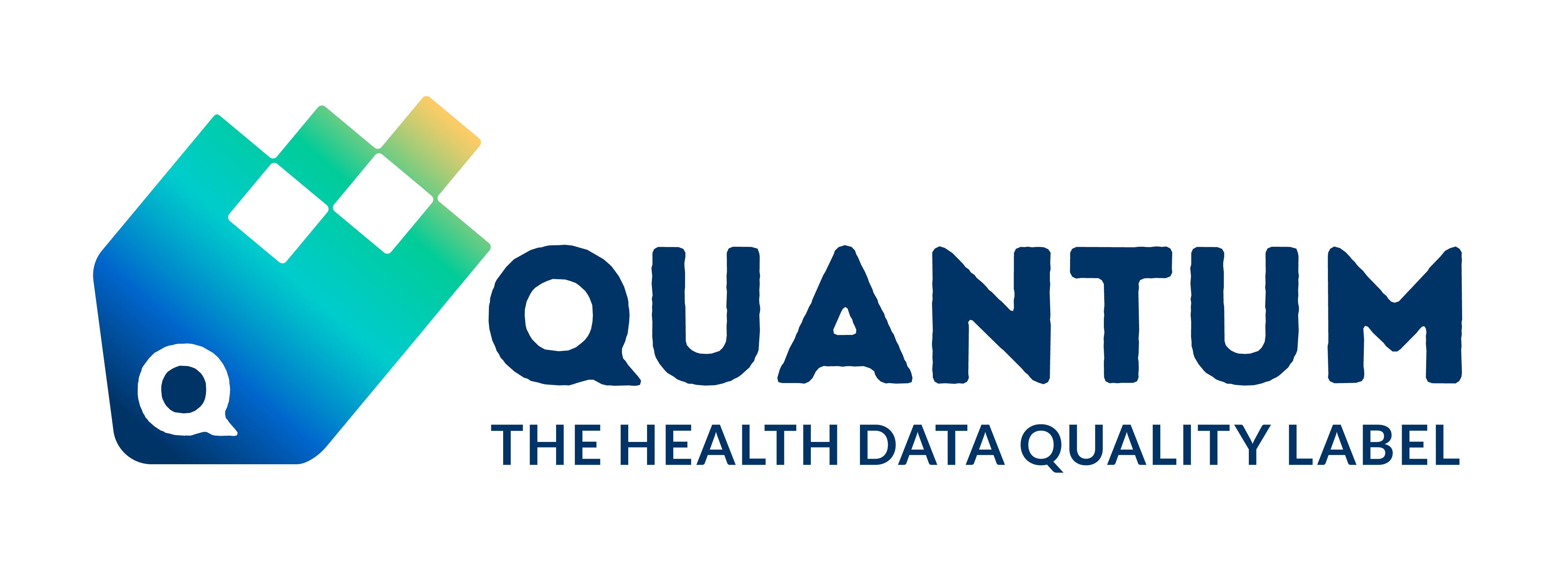
Are you a data holder or a data user? Find here precious resources about data quality. As an exclusive feature for the European Health Information Portal, this page gives you access to all you need to know about Data Quality, bringing to you the main insights from the QUANTUM project.
Why data quality is important?
The quality of both data and datasets is vital for the secondary use of health information (for research, innovation and policymaking). High-quality datasets enhance the reliability, validity, and ethical integrity of public health research, supporting better decision-making and ultimately improving health outcomes. Data quality refers to how well data fits the user’s needs. The main features of data quality are relevance, accuracy and reliability, coherence, coverage, completeness and timeliness.
In the context of the European Health Data Space (EHDS), the Joint Action Towards the European Health Data Space (TEHDAS) has developed a framework to identify the main elements of data quality and utility in the European health data space (EHDS), and has made recommendations to the European Commission and the member states on data quality and utility in the EHDS. Read the TEHDAS 13 recommendations for ensuring the quality and utility of data in the EHDS here.
About Quantum:
QUANTUM is an EU-funded project (2023-2025) that aims to create a common label system for Europe that guarantees the quality and utility of datasets* for scientific and health innovation purposes. This label system will enable researchers, policymakers, and healthcare professionals to identify high-quality data for research and decision making. QUANTUM will address Article 56 of the European Health Data Space (EHDS) regulation*, which mandates labelling health datasets to show their quality and usefulness for secondary use of health data. Visit www.quantumproject.eu to learn more!
Out of this project, many key insights, reflections, and resources are made available on this page for you about data quality.
*What do we call a dataset?
A Dataset is a collection of data, published or curated by a single source, and available for access or download in one or more formats. In the context of the EHDS Regulation [EUR-Lex - 52022PC0197 (Art.44)], access to such datasets must adhere to principles of data minimisation and purpose limitation, ensuring that only the data relevant and necessary for the specified processing purpose is provided, in either anonymised or pseudonymised format depending on the feasibility of achieving the processing objectives.
Examples of datasets:
- A simple dataset: A single database, a CSV file etc.
- A complex dataset: A set of three databases related to a specific cohort of cancer patients:
- relational database of the patient’s medical history;
- a set of the patients’ cancerous cell images;
- the logs of the microscope used to generate these images at the moment of the examination.
These three databases can be used together via shared identifiers (patient identifier in medical history & cancerous cell images, images belonging to batches whose identifier can be found in both images & microscope logs).
*What exactly does Article 56 of the European Health Data Space regulation say?
Article 56 - Data quality and utility label
- Datasets made available through health data access bodies may have a Union data quality and utility label provided by the data holders.
- Datasets with electronic health data collected and processed with the support of Union or national public funding shall have a data quality and utility label, in accordance with the principles set out in paragraph 3.
- The data quality and utility label shall comply with the following elements:
- (a) for data documentation: meta-data, support documentation, data model, data dictionary, standards used, provenance;
- (b) technical quality, showing the completeness, uniqueness, accuracy, validity, timeliness and consistency of the data;
- (c) for data quality management processes: level of maturity of the data quality management processes, including review and audit processes, biases examination;
- (d) coverage: representation of multi-disciplinary electronic health data, representativity of population sampled, average timeframe in which a natural person appears in a dataset;
- (e) information on access and provision: time between the collection of the electronic health data and their addition to the dataset, time to provide electronic health data following electronic health data access application approval;
- (f) information on data enrichments: merging and adding data to an existing dataset, including links with other datasets;
- The Commission is empowered to adopt delegated acts in accordance with Article 67 to amend the list of principles for data quality and utility label. Such delegated acts may also amend the list set out under paragraph 3 by adding, modifying or removing requirements for data quality and utility label.
- The Commission shall, by means of implementing acts, set out the visual characteristics and technical specifications of the data quality and utility label, based on the elements referred to in paragraph 3. Those implementing acts shall be adopted in accordance with the advisory procedure referred to in Article 68(2). Those implementing acts shall take into account the requirements in Article 10 of Regulation […] [AI Act COM/2021/206 final] and any adopted common specifications or harmonised standards supporting those requirements
QUANTUM's interesting insights on Data quality:
1. Conceptualisation of data quality & utility: study on data quality terms and dimensions
In order to better understand how various definitions and dimensions of data quality are perceived by professionals and organizations involved in health data management, the QUANTUM project conducted a study in the format of a a comprehensive survey. The participants, representing a diverse range of roles such as data users, data holders, data quality managers, and data analysts, came from healthcare institutions, public health agencies, and research networks across Europe.
Main findings from the QUANTUM study on data quality terms and dimensions.
-
The survey explored key terms like "fit for purpose," "fit for use," "data quality," and "data utility," among others, to assess the level of agreement or disagreement among respondents regarding their definitions. The findings indicate a considerable consensus on many of these definitions, suggesting a common understanding of fundamental data quality concepts within the community. For example, there was broad agreement on the definitions of "accuracy," often described as the closeness of data to its true value, and "completeness," which refers to the degree to which all necessary data is present.
However, the study also revealed some differences in interpretation, particularly with more nuanced concepts such as "data utility" and "provenance." These differences underscore the complexity and context-dependent nature of these terms. The term "uniqueness," for instance, was interpreted in various ways, with some respondents focusing on the non-duplication of data and others emphasising the minimisation of redundancy.
The survey also highlighted the importance of certain data quality dimensions over others, depending on the specific roles or organizational needs of the respondents. Provenance and traceability emerged as particularly critical dimensions, especially in contexts where maintaining data lineage and transformation history is vital for ensuring data integrity and trustworthiness.
In conclusion, the study reveals both a consensus and diversity in the understanding and prioritisation of data quality dimensions among health data professionals. This insight is crucial for developing standardised quality labelling tools or frameworks that can address varying interpretations while ensuring high data integrity and usability across different contexts. The findings will inform the creation of a more universally accepted and applied data quality labelling system, particularly in public health and research settings.
2. Landscape analysis of existing data quality related technologies and tools
One of the first tasks of the QUANTUM project, in order to determine the best options for creating a data quality labelling tool, was to perform a landscape analysis of existing tools and technologies, meaning tools that provide measurements for data quality dimensions, have an open-source license, and can apply to health data. Find below interesting findings from this analysis.
Main findings from the QUANTUM landscape analysis on existing tools related to data quality
Identified tools
- From known literature: Tools like OHDSI Achilles, Data Cleaner, Pentaho Kettle, Apache Griffin, and MobyDQ were identified.
- From systematic review: Focused on articles from the last ten years in English. Tools such as BUSCO, GTQC, and IeDEA Harmonist Data Toolkit were found.
- From AI search: AI tools highlighted Great Expectations, OHDSI Data Quality Dashboard, MobyDQ, and others.
- From partner survey: the survey reached 22 responses from QUANTUM partners and 5 from external entities. Commonly used tools include R and Python packages, OHDSI suite, Pentaho, and Apache Superset.
Exploration
Four specific tools were further examined for their capabilities:
- Great expectations: A Python library for creating and validating data quality checks.
- DQOps: Software with a graphical interface for configuring and executing data quality assessments.
- R Scripting: Custom R scripts for flexible data quality assessments.
- OHDSI Data Quality Dashboard: An R package that applies standardized data quality checks in the OMOP model.
To integrate and share the results of the data quality labeling tool, the QUANTUM project must consider interoperability standards. These include TEHDAS, EHDS2, DCAT, and DQV vocabularies, which help ensure data can be shared and understood across different systems.
The project also explored several visualization tools to display data quality labels and gather user feedback:
- Shields.io: Creates badges representing data quality.
- Open Badges: Developed by Mozilla, used for validating accomplishments.
- FairPlus: Measures the FAIRness (Findable, Accessible, Interoperable, Reusable) of data.
Design options for Input were also explored:
- Self-assessment form: Data holders manually input measurements.
- API input: Programmatic data entry via an Application Programming Interface.
- Automatic mapping: Data holders’ existing tools generate results that the QUANTUM tool maps into a standard format.
Conclusion
The analysis shows a broad range of data quality tools available, many of which are mature and support custom metrics and exportable results. This supports the development of a non-invasive, interoperable QUANTUM data quality labelling tool.
The landscape analysis reveals a heterogeneous set of tools suitable for developing the QUANTUM labeling tool. Most tools allow custom metrics and export results in parsable formats. Future work will finalize the tool's design and integration with data holders and access bodies.
What existing tools are actually used in the health research field? Results from the QUANTUM stakeholders' survey
As part of the above landscape analysis, a comprehensive survey was conducted among QUANTUM partners and external experts to understand the tools and methodologies currently in use for measuring data quality and utility. This is a specific interest, as all the respondents are specifically in the health sector, being either data users or data holders. Their responses are thus specifically relevant to be highlighted, as it illustrates the current sate of the art in the health information field. Find below the key findings of the survey:
Respondent Demographics
- Total responses: 22 responses from QUANTUM partners and 5 responses from external entities.
- Countries represented: Various European countries contributed to the survey.
Secondary Uses of Data
- Most common use: Research (28% of responses)
- Other uses: Healthcare professionals' capacity building and training, Artificial Intelligence, Clinical quality improvement, Planning and management of health and social care services, and Clinical quality assurance.
Categories of Data
- EHDS Article 33 categories: Data impacting health, Electronic health data from medical registries for specific diseases, Electronic Health Records (EHRs).
Data Types
- Most common data type: Structured/semi-structured data.
- Other data types: Text (e.g., patient notes), Imaging (e.g., DICOM), Omics data (e.g., genomic sequences).
Tools used for data quality measurement
- Usage statistics: 66% of respondents use existing or in-house developed tools, 18% are considering or in the process of using a tool, and 15% do not use any tools.
- Popular tools:
- Programming languages like R and Python (custom scripts and packages)
- Specific software solutions like Talend, Apache Airflow, Pentaho, Microsoft Excel, PowerBI, Grafana, IBM Cognos Analytics, Eurostat Metadata Handler, and OHDSI suite (Achilles, Rabbit in a Hat, Athena, Data Quality Dashboard)
- Manual procedures like data quality questionnaires and the HIQA Data Quality Assessment Tool
Custom Metrics and Export Capabilities
- Custom Metrics: 75% of respondents confirmed that their tools allow the definition of custom metrics.
- Export Capabilities: 80% of respondents' tools can export results in external or parsable files.
This detailed information from the survey results can guide the development and design of the QUANTUM data quality labelling tool, ensuring it meets the needs of various stakeholders and integrates seamlessly with existing data management practices.
Join the QUANTUM academy!
In order to build capacity within the public health community about data quality, QUANTUM is about to launch its academy! Stay tuned for more information.
Any question? Contribution? Contact us!
If you have any question about the content that is presented here, or if you have relevant valuable content that you would like to be highlighted here, do not hesitate to contact us through the button below.